Middle English Translator
I trained the first ever neural model for English to Middle English translation.
Neural Machine Translation
I wanted to do a project with neural Machine Translation and a low-resorce language. Low-resource languages are languages that don't have an abundance of written text
and often don't enjoy the prestige of high-resource languages like English, Mandarin, and German. They can often suffer from lack of support by governments and educational systems.
Speakers of low-resource languages are also at a disadvantage because most technologies are not translated in their language. (you can't talk to your Alexa in Mapuche, for example.)
The last neural Machine Translation project I did was in 2018 before large transformer-based language models had really become ubiquitous. Since the technology has improved, I figured I can improve.
There's a problem with my plan, however. I don't speak or understand any low-resource languages.
To get around this issue, I decided to simulate a low-resource language using Middle English, a variety of English spoken from the 11th-15th centuries CE. There are a number of surviving texts, but not a ton.
Middle English is also a good target because it's very similar to modern English, which should make this somewhat easier.
Middle English
There are a number of common misunderstandings about the history of English. At the beginning of my Linguistics degree, I was surprised to learn that what I always thought of as 'Old English' wasn't old English at all. Scholars divide the history of the English language into 4 categories:
- Modern English
- Early Modern English
- Middle English
- Old English
If you're reading this post, chances are you speak Modern English, so we're not going to cover it here.
One common illustration of these different varieties of English is by reproducing the Lord's Prayer.
Early Modern English:
Our father which art in heauen,
hallowed be thy name.
Thy kingdome come.
Thy will be done, in earth, as it is in heauen.
Giue vs this day our daily bread.
And forgiue vs our debts,
as we forgiue our debters.
And lead vs not into temptation,
but deliuer vs from euill
Middle English:
Oure fadir that art in heuenes,
halewid be thi name;
thi kyndoom come to;
be thi wille don in erthe as in heuene:
gyue to us this dai oure breed ouer othir substaunce;
and forgyue to us oure dettis,
as we forgyuen to oure gettouris;
and lede us not in to temptacioun,
but delyuere us fro yuel.
Old English:
Fæder ure þu þe eart on heofonum;
Si þin nama gehalgod
to becume þin rice
gewurþe ðin willa on eorðan swa swa on heofonum.
urne gedæghwamlican hlaf syle us todæg
and forgyf us ure gyltas
swa swa we forgyfað urum gyltendum
and ne gelæd þu us on costnunge
ac alys us of yfele
As we go back in time the language gets harder to understand, with Old English basically unintelligable to modern people.
Getting the data
In order to train a neural translation model, you need a bunch of data. This data, ideally, should be in the form of sentences paired with their translations.
As I mentioned earlier, Middle English is something of a low-resource language. Whereas languages like Spanish have millions of web resources easily available, there are at most a few hundered surviving books in Middle English.
Thankfully, we still have a couple of great resources for paired text: the Wycliffe Bible and Geoffery Chaucer's Canterbury Tales.
These are both good resources because they're very long and have Modern English translations. Scholars have produced line-by-line translations of the Canterbury Tales, and the Wycliffe Bible can be easily aligned verse-by-verse with a modern English version of the Bible.
In all, the training data for this model includes about 60,000 paired sentences, taken from Chaucer's complete works, the Wycliffe Bible, and Sir Gwain and the Green Knight.
I wrote a few python scripts to scrape this data from the internet and align the sentence pairs. The dataset is available here.
Training a Model
As readers of this blog know, I tend to tackle most of my problems using large language models. This translator is no exception.
Large lanugage models allow us to leverage the underlying probability distribution of language.
We then use that probability distribution as a prior before fine-tuning the model on a specific task. (in this case, translation to Middle English)
For the translator we use BART, a denoising language model created by Facebook in late 2019. Training the model is straightforward.
In essence, we show it a sentence in English, and then show it the Middle English translation. Every so often, we test the model by asking it to translate some sentences it hasn't seen before. We use that test to see how much the model is learning.
At the end of training, we test the model using a metric called BLEU (a measure of machine translation goodness) on another batch of sentences it hasn't seen before.
This model achieves a BLEU score of about 17 (not great), but the score is artificially deflated by features of Middle English such as spelling inconsistencies and the irregular grammar of a changing language.
Testing the Translator
While the BLEU score gives us some idea of how good our translator is, the best evaluation method is testing it out.
You can try the demo here.
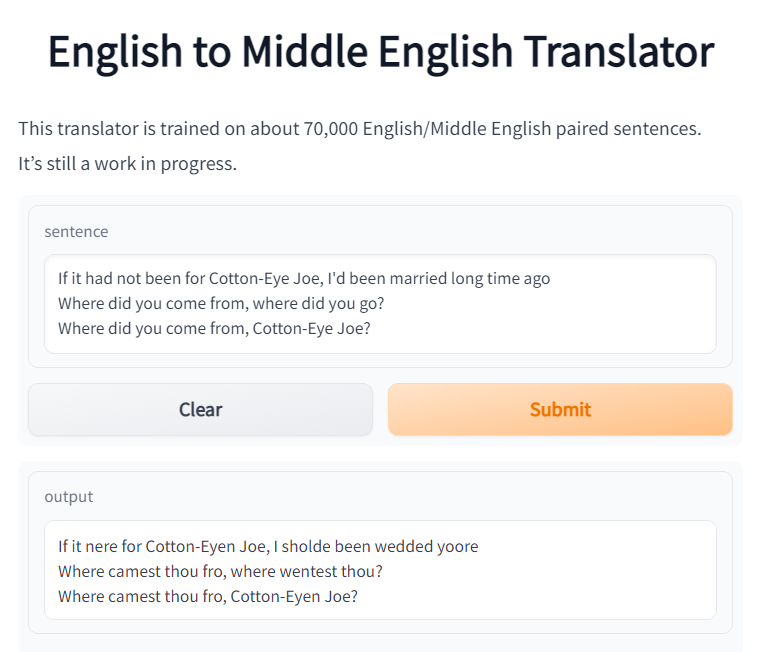
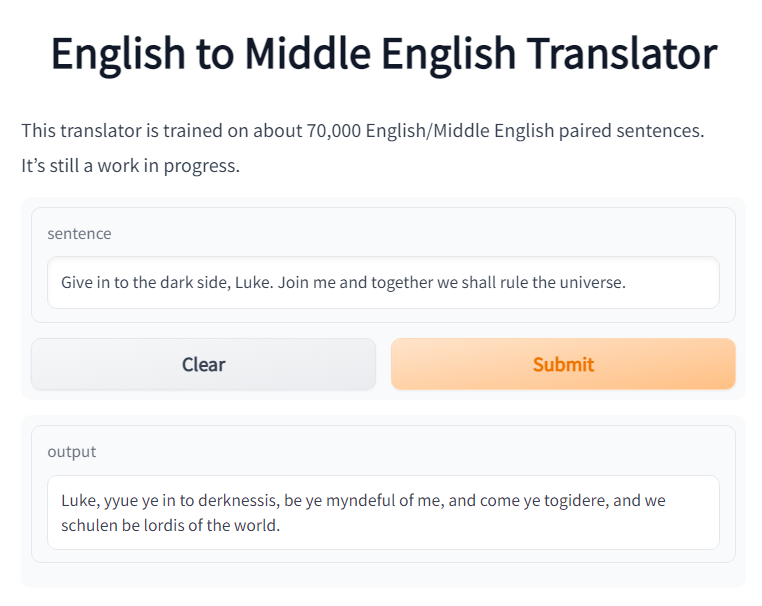
Middle English was different from Modern English in more ways than just spelling and vocabulary. There were substantive differences in grammar, as well. Middle English handled negation differenly from Modern English using something called a negative concord. For example, note the following line from Chaucer's Canterbury Tales:
He nevere yet no vileynye ne sayde
(He never said anything rude)
Middle English also had certain morphological characteristics lost in Modern English. For example, verbs changed their structure.
Whereas we would put the past tense of the verb help as helped, Middle English would change the vowel and the ending to holpen.
The model has learned both of these features.
Here's an example:
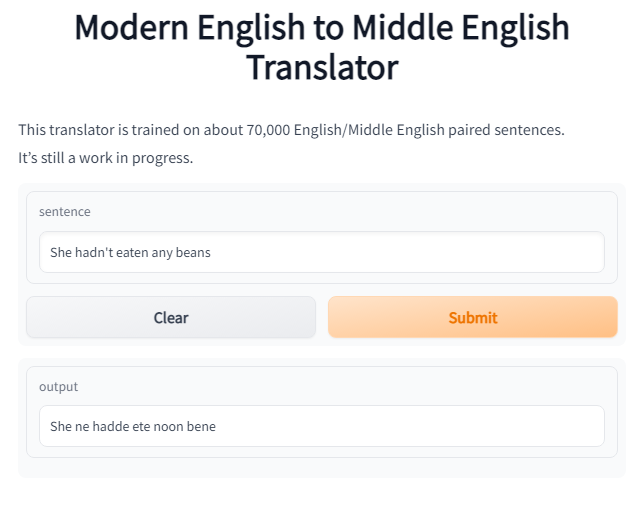
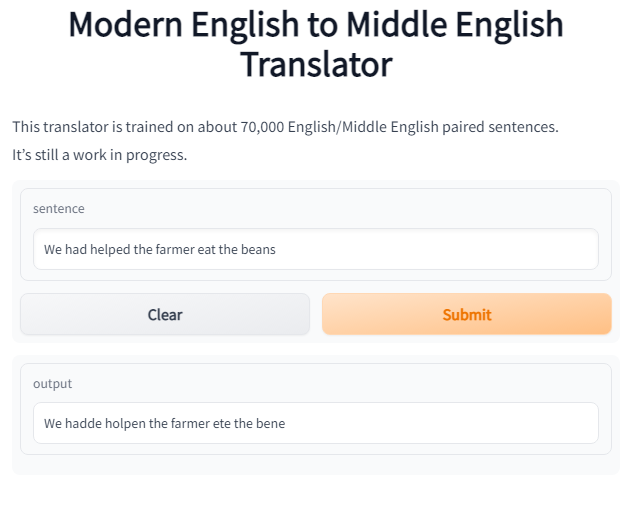
Limitations
This model is limited by the training data. Because at least half of the data comes from poetry, the model is very good at translating poetry.
Here's an example from a beloved children's book, The Cat in the Hat:
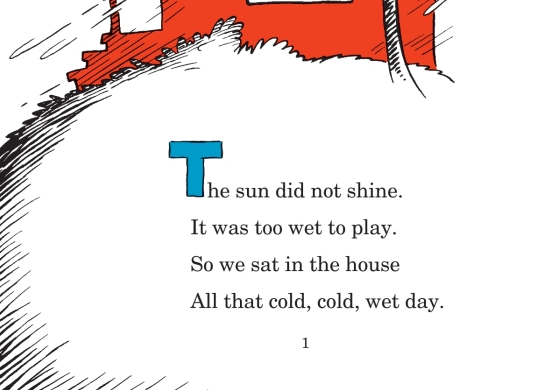
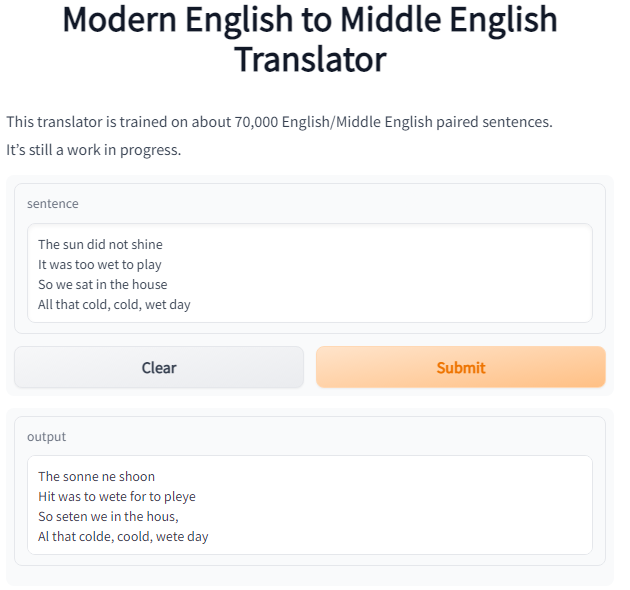
However, it struggles with certain types of prose such as commands and reported dialog.
The model also has difficulty with vocabulary that doesn't often occur in the training set.
Finally, as mentioned earlier, Middle English is not a monolith. Spelling varies from paragraph to paragraph and different regions had vastly different vocabularies.
This hurts the translator because if it sees the word egg translated as ei, aye, egge, ey and heye with plurals including egges, eyrene, eirne, eirene, and hegges,
the translator can't learn a good one-to-one mapping.
Conclusion
In spite of its limitations, the English to Middle English translator was a rousing triumph. I shared it on Reddit, and more than 500 people saw it and liked it. More importantly, I was able to work on a neural Machine Translation project and I learned a lot.